Investigating the Stability of Job Importance and Job Satisfaction over Time: A Latent Transition Analysis of the Survey of Doctorate Recipients
Disclaimer
Working papers are intended to report exploratory results of research and analysis undertaken by the National Center for Science and Engineering Statistics (NCSES). Any opinions, findings, conclusions, or recommendations expressed in this working paper do not necessarily reflect the views of the National Science Foundation (NSF). This working paper has been released to inform interested parties of ongoing research or activities and to encourage further discussion of the topic and is not considered to contain official government statistics.
This research was completed while Dr. Fritz was on academic leave from the University of Nebraska–Lincoln and participating in the NCSES Research Ambassador Program (formerly the Data Analysis and Statistics Research Program) administered by the Oak Ridge Institute for Science and Education (ORISE) and Oak Ridge Associate Universities (ORAU). Any opinions, findings, conclusions, or recommendations expressed in this working paper are solely the authors’ and do not necessarily reflect the views of NCSES, NSF, ORISE, or ORAU.
Abstract
Previous applications of person-centered approaches to investigate job satisfaction and job importance using the Survey of Doctorate Recipients (SDR) have focused on describing cross-sectional relations between these variables within a collection cycle. The working paper presented here expands this previous research to explore whether (and if so, how) doctoral degree recipients transition between job satisfaction classes over time using latent transition analysis. The results show that more than half of the doctoral degree recipients in the sample transitioned to a different job satisfaction class at some point during the 2015, 2017, and 2019 SDR cycles. Notably, among respondents who transitioned to a new job satisfaction class between the 2015 and 2017 surveys, those who reported in 2015 being very satisfied with their current job’s level of independence, challenge, and responsibility but only moderately satisfied with their job’s salary and benefits in 2015 were most likely to report being only somewhat satisfied with all factors of their current job at the 2017 cycle. In addition, individuals who reported being only somewhat satisfied with all factors of their current job in 2017 and transitioned to a new job satisfaction class in 2019 were more than twice as likely to report being dissatisfied with their opportunities for advancement at the 2019 cycle than being very satisfied with all factors of their job in 2019. This finding indicates a potential pathway by which individuals who were initially very satisfied with at least some factors of their current job become dissatisfied. The results also showed that the probability of changing job satisfaction classes differed depending on an individual’s job importance class membership, employment sector, and self-reported race and ethnicity. Conclusions based on these results, as well as limitations and future directions for this research, are discussed.
Introduction
Background
The working paper presented here is the third in a series of working papers (Fritz 2022a, 2022b) that has used person-centered approaches to model job importance and job satisfaction for doctoral degree recipients using the Survey of Doctorate Recipients (SDR; NCSES 2021). This series of working papers serves two purposes. First, it provides insight into what is fueling the growing trend for academic science, technology, engineering, and mathematics (STEM) researchers to transition to nonacademic careers (Ruiz Castro, Van der Heijden, and Henderson 2020). An engaged and productive cadre of academic STEM researchers is vital to the health of a country’s science and engineering enterprise, not just to train the next generation of scientists and engineers but also to increase scientific knowledge and promote innovation through basic research, applied research, and university-industry collaborations (Kaloudis et al. 2019). A better understanding of the job factors that academic STEM researchers value, their satisfaction with each of these factors in their academic jobs, and how these two constructs relate and change over time could help identify the tipping point at which a researcher decides to leave academia. This scenario in turn would allow universities to intervene to potentially mitigate or reverse this so called “academic brain drain” (Odhiambo 2013).
The second purpose of this working paper series is to illustrate how person-centered approaches, which may be unfamiliar to researchers of the science and engineering enterprise and the science and engineering workforce, can reveal relationships and trends in data that more traditional variable-centered approaches cannot. Variable-centered approaches, such as analysis of variance and multiple regression, are designed to test for differences between observed groups (e.g., degree area) on a specific variable or to model the relationship between two continuous variables when controlling for other variables. In contrast, person-centered approaches, such as latent class analysis (LCA; see Fritz 2022a for more information), are designed to discover unobservable groups based upon patterns of responses to a set of variables. Because attitudes and beliefs such as job satisfaction are multifaceted, often measured using multiple survey questions, and can change over time, person-centered approaches offer several advantages over variable-centered approaches when exploring how job satisfaction and job importance are related over time.
Fritz (2022a) conducted an LCA of doctoral degree recipients’ ratings of the importance of and their satisfaction with nine job factors using the 2017 SDR cycle data (NCSES 2019). The results suggested that doctoral recipients belonged to one of five job importance classes: (1) Everything Is Very Important, (2) Challenge and Independence are More Important than Salary and Benefits, (3) Benefits and Salary Are More Important than Responsibility, (4) Everything Is Somewhat Important, and (5) Advancement, Security, and Benefits Are Unimportant and one of five job satisfaction classes (1) Very Satisfied with Independence, Challenge, and Responsibility, (2) Very Satisfied with Everything, (3) Very Satisfied with Benefits, (4) Dissatisfied with Opportunities for Advancement, and (5) Somewhat Satisfied with Everything. Further analysis found small but potentially important differences in class membership based on self-reported race and ethnicity, age and years since doctorate, employment sector, and workforce status as well as a general lack of concordance between job importance class and job satisfaction class. Fritz (2022b) then extended these results by showing that the five-class LCA solutions for job importance and job satisfaction found in the 2017 SDR cycle replicated in the 2015 and 2019 SDR cycles.
As noted in Fritz (2022b), the replication provides strong evidence that the same job importance and job satisfaction classes exist at all three SDR waves. The replication does not, however, directly test any specific longitudinal hypotheses such as whether class membership is stable over time or, if not, how doctorate recipients transition between classes. Given the goal of identifying what motivates an academic researcher to leave academia, the primary focus of the working paper presented here is to extend the results of the prior working papers to determine who is most likely to transition into the Dissatisfied with Opportunities for Advancement class. A secondary goal is to examine whether job importance class membership or participant characteristics, such as employment sector, influence these transitions.
This working paper extends the results from Fritz (2022a, 2022b) in three important ways. First, this working paper uses latent transition analysis (LTA) to determine the level of stability of the latent job satisfaction and job importance classes over time and, for those who transition to different classes, the likelihood of transitioning to a specific class at the next SDR cycle. Second, this working paper uses associative LTA (ALTA) to test whether the likelihood of transitioning between job satisfaction classes differs based on job importance class membership. Finally, this working paper uses LTA with covariates to explore whether the likelihood of transitioning to a different job satisfaction class depends on one or more of a participant’s demographic characteristics.
Methods
Data
The data for this working paper come from the restricted-use microdata files for the 2015, 2017, and 2019 cycles of the SDR (NCSES 2018, 2019, 2021, respectively). Because this paper focuses on examining change between cycles (and because the 2019 cycle dropped individuals who did not respond to both the 2015 and 2017 cycles while adding 14,564 individuals who were eligible but not selected for the 2015 cycle), the decision was made to include only individuals who completed the 2015 cycle questionnaire. Inclusion criteria for the 2015 SDR cycle included a completed doctoral research degree from a U.S. academic institution prior to 1 July 2013, being less than 76 years of age on 1 February 2015, and not being institutionalized or terminally ill at the time of data collection.
Participants
A total of 78,286 doctoral recipients completed the job importance questions for the 2015 SDR cycle, with 88.5% (n = 69,317) also completing the job importance questions for the 2017 or 2019 cycle and 65.6% (n = 51,383) completing the job importance questions for all three cycles. As shown in table 1, the majority of participants identified as male (60.1%), White (63.0%), and as not having a physical disability (90.5%) during the 2015 cycle. The participants were also predominately between the ages of 30 and 59 (71.7%), held a doctoral degree in a science field (78.5%), and were living in the United States during the week of 1 February 2015 (86.7%). Only SDR participants who were employed the week of data collection were asked about their job satisfaction. In total, 89.8% (n = 70,303) of the 2015 SDR cycle participants completed the job satisfaction questions at least once during the 2015, 2017, and 2019 cycles. Of those 70,303, 59.6% (n = 41,932) completed the job satisfaction questions for all three cycles, 25.7% (n = 18,041) completed the job satisfaction questions for two of the cycles, and the remaining 14.7% (n = 10,330) completed the job satisfaction questions for a single cycle.
Survey of Doctorate Recipients participant characteristics: 2015
Note(s):
The values presented here are provided for reference only because they are based on the reduced sample used for the current project and therefore do not match the official values reported by the National Center for Science and Engineering Statistics. All participants who identified as Hispanic were included in the Hispanic category, and only in the Hispanic category, regardless of whether they also identified with one or more of the racial categories. The "other" category included individuals who identified as more than one race.
Source(s):
National Center for Science and Engineering Statistics, Survey of Doctorate Recipients.
Survey Questions
This working paper focuses on two SDR survey questions, C6 and A34. Question C6 asked participants to rate the importance of the following nine job factors as “very important,” “somewhat important,” “somewhat unimportant,” or “not important at all:” (1) salary, (2) benefits, (3) job security, (4) job location, (5) opportunities for advancement, (6) intellectual challenge, (7) level of responsibility, (8) degree of independence, and (9) contribution to society. Question A34 asked participants who reported being employed the week of data collection to rate their satisfaction with the same nine job factors for their current job as “very satisfied,” “somewhat satisfied,” “somewhat dissatisfied,” or “very dissatisfied.” As in the two preceding working papers (Fritz 2022a, 2022b), “somewhat unimportant” and “not important at all” were combined into a single “unimportant” category and “somewhat dissatisfied” and “very dissatisfied” were combined into a single “dissatisfied” category, resulting in three response options for each question. Estimated population frequencies for the three-response data for the 2015, 2017, and 2019 cycles are shown in Fritz 2022b: table 2.
Analyses and Software
Note that this section contains a detailed description of the statistical models and software used in this analysis. Readers less interested in this technical information may wish to examine images 1 and 2, and then skip to the Results section.
Latent Transition Analysis
The main form of analysis used for the current working paper is LTA, which extends the cross-sectional LCA model to allow individuals to transition between classes across time. Specifically, this paper extends the five-class, three-response LCA model for the nine job factors found by Fritz (2022a, 2022b)
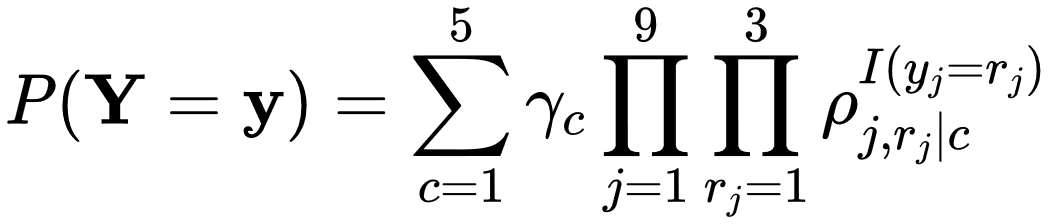
|
(1) |
to allow individuals to transition between latent classes for the 2015, 2017, and 2019 SDR cycles such that

|
(2) |
where γc15 is the probability of membership in a specific class in 2015 (i.e., class prevalence in 2015), τc17|c15 is the probability of being a member of a specific class in 2017 given membership in a specific class in 2015 (i.e., class transition probability for 2015 to 2017), τc19|c17 is the probability of being a member of a specific class in 2019 given membership in a specific class in 2017 (i.e., class transition probability for 2017 to 2019), and ρj,rj,t|ct is the probability of a participant rating job factor j as response option r at cycle t given their membership in a specific class c at cycle t (i.e., item-response probability). The three-wave LTAs for job satisfaction and job importance (modeled separately) are illustrated in image 1. For more information on the LCA and LTA mathematical models, see Fritz (2022a, 2022b) or Collins and Lanza (2010).
Although Fritz (2022b) found that the five-class LCA solutions for job importance and job satisfaction for the 2017 SDR cycle replicated in the 2015 and 2019 cycles, there was some slight variability in the item-response probabilities across cycles. This variability is likely due to sampling error but could complicate the interpretations of the transition probabilities in the LTA models. In order to ensure the latent classes are identical across cycles, Collins and Lanza (2010) recommend imposing longitudinal measurement invariance, which is achieved by freely estimating the item-response probabilities using the data at all measurement occasions simultaneously while constraining the time-specific item-response probabilities (i.e., ρj,rj,t|ct) to be equal across measurement occasions. The transition probabilities are not constrained to be equal across time, however, as differences in the 2017 to 2019 transition probabilities compared to the 2015 to 2017 transition probabilities are of theoretical interest. Collins and Lanza also recommend handling missing data in LTA models using full information maximum likelihood, which allows cases with missing observations (e.g., someone who participated in the 2015 and 2019 cycles but not the 2017 cycle) to still contribute information to the final model. The decision was also made not to include the SDR sampling weights in any of the LTA models based on four factors:
- a lack of clarity with regard to the competing recommendations for which sampling weights should be used for longitudinal models when separate weights are calculated for each cycle (or even whether sampling weights should be used at all in regression models),
- the available computational resources for fitting these very computationally intensive models (more on this in the next section),
- a desire to be conservative with regard to both the generalizability of the findings and the type I error rates for statistical tests of significance in a very large sample, and
- exploratory LTA models fit with sampling weights being similar to the final models that did not include sampling weights.
As a result, the findings presented here describe the sample and should not necessarily be generalized to the population.
Associative Latent Transition Analysis
In addition to understanding how participants transition between classes for a single discrete process, this working paper seeks to determine whether participant characteristics, such as job importance class or employment sector, can predict transitions in job satisfaction class. Modeling two or more discrete, latent processes simultaneously as well as the interrelations between those processes can be accomplished using ALTA (Bray, Lanza, and Collins 2010; Flaherty 2008). There is a temptation to treat the ALTA models, which are loglinear models with latent variables (Hagenaars 1993), as simply latent variable versions of autoregressive panel models. For example, combining the separate LTA models for job importance and job satisfaction in image 1 and adding lags and cross-lags between variables at will, such as in the cross-lagged ALTA model shown in image 2, part A. This approach has two challenges, however. First, the LTA model for a single process is a complex model on its own and becomes multiplicatively more intricate when examining the relations between multiple processes. This point is emphasized when attempting to fit a cross-lagged ALTA model using all three cycles of the SDR data, as the model would not run from the computational requirements to estimate the model exceeding those available. Second and more importantly, the question is not whether job importance class at one cycle predicts job satisfaction class at the next cycle or vice versa, but whether job importance class can predict the probability of transitioning between job satisfaction classes across time. In other words, there may be an interaction between job satisfaction and job importance such that the job satisfaction transition probabilities differ by job importance class.
To test whether there is an interaction, Flaherty (2008) describes an ALTA model where one of the latent class variables is designated as the outcome variable D and the other represents the predictor C. Each measurement of D is then regressed onto C at the same measurement occasion, both C and D at the previous measurement occasion, and the interaction between C and D at the previous measurement occasion. Using Collins and Lanza’s (2010) notation, the equation for Flaherty’s ALTA model with T waves of data is as follows:
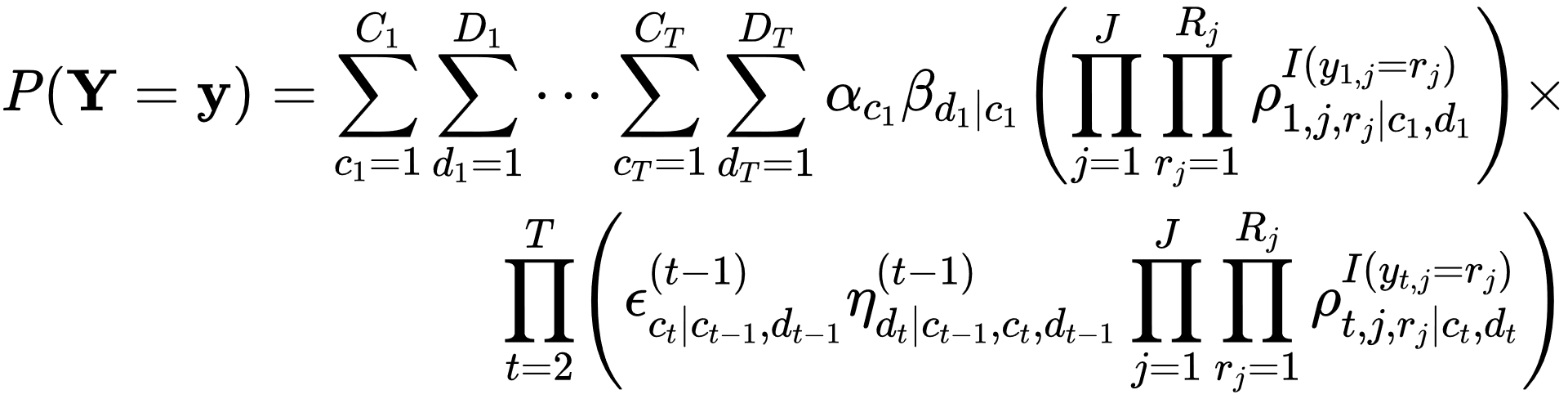
|
(3) |
where αc1 is the probability of belonging to a specific class of C at the first measurement occasion, βd1|c1 is the probability of belonging to a specific class of D at the first measurement occasion conditional on membership in a specific class of C at the first measurement occasion, εct|ct-1,dt-1 is the probability of belonging to a specific class of C at measurement occasion t conditional on membership in specific classes of C and D at measurement occasion t – 1, ηdt|ct-1,ct,dt-1 is the probability of belonging to a specific class of D at measurement occasion t conditional on membership in specific classes of C and D at measurement occasion t – 1 and C at measurement occasion t, and ρ is the measurement occasion-specific item-response probabilities. As desired, this model will output separate matrices of transition probabilities for D for each class of C. Image 2, part B shows Flaherty’s general ALTA model with job satisfaction as the dependent variable and job importance as the predictor with the solid arrows representing the main effects and the dashed arrows representing the interaction terms.
Given the computational resource issues encountered with attempting to fit the three-wave cross-lagged ALTA model, two separate two-wave ALTA models were estimated, one for the 2015 to 2017 cycle transitions and a second for the 2017 to 2019 transitions, with job importance at the second wave not included. Note that by fixing the item-response probabilities for job satisfaction and job importance to the values found in their respective individual LTA models, the impact of estimating these models separately should be minimal. The two-wave 2015 to 2017 ALTA model is shown in image 2, part C and described by the following equation:
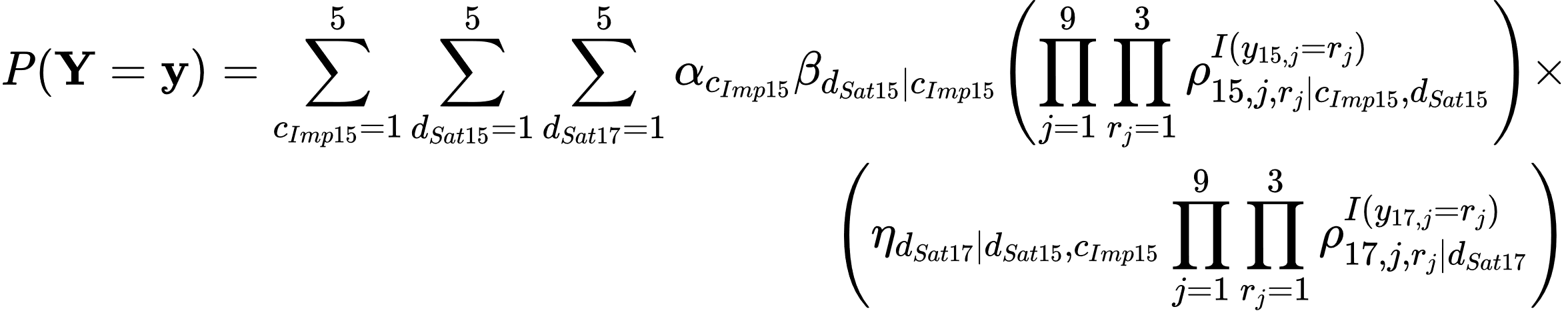
|
(4) |
As noted by Flaherty (2008), this simplified version of the ALTA model can be estimated by setting a series of constraints to remove specific unwanted effects from the model.
An advantage of conceptualizing the ALTA model in this way is that because equations 3 and 4 are just a set of simultaneously estimated multinomial logistic regressions, the model with the interaction terms constrained to be zero (i.e., without the dashed arrow) is nested within the model in which the interaction terms are freely estimated (i.e., with the dashed arrow), so the difference in model fit between these two models can be tested statistically and acts as an omnibus test of the interaction terms. Because the fit of multinomial logistic regression models requires a scaled (i.e., mean-adjusted) chi-square, testing the difference in fit requires a Satorra-Bentler chi-square difference test for scaled chi-squares (Satorra and Bentler 2010) given as
|

|
(5) |
L0 is the loglikelihood of the model with the interaction terms constrained to be zero, L1 is the loglikelihood of the model with the interaction terms freely estimated, s0 is the scaling correction for the constrained model, s1 is the scaling correction for the unconstrained model, p0 is the number of parameters in the constrained model, and p1 is the number of parameters in the unconstrained model. The significance of χ2ΔSB is determined by comparing this value to a chi-square distribution with degrees of freedom equal to the number of parameters associated with the interaction terms (i.e., p1 – p0; Agresti 2013).
Latent Transition Analysis with Covariates
Another advantage of fitting the model in image 2, part C is that the impact of manifest covariates, such as gender or employment sector, on the job satisfaction transitions can be examined simply by replacing job importance class with the desired covariate. Based on this scenario, separate two-wave ALTA models were fit to investigate the effects of gender, physical disability, race and ethnicity, degree area, U.S. residency at the time of data collection, and employment sector on the transitions for job satisfaction class.
Software
The restricted-use microdata files for the 2015, 2017, and 2019 SDR cycles were accessed through the NORC Data Enclave (NORC at the University of Chicago 2016). SAS version 9.4 was used to merge the data for the separate cycles and to compute descriptive statistics. All LTA and ALTA models were estimated with Mplus 8.7 using the default estimator for the MIXTURE command (maximum likelihood with robust standard errors; MLR) and 200 random starts, 100 of which were carried through all three estimation stages (STARTS ARE 200 100 100). The nine job factors were treated as ordered categorical variables using the CATEGORICAL command and missing data were addressed using full information maximum likelihood; as recommended by Mplus, the observed demographic variables (e.g., gender) were included in the ALTA models using the KNOWNCLASS command. The ALTA models were specified by regressing job satisfaction class at the second wave on both job satisfaction class and the predictor variable at the first wave as well as regressing job satisfaction class at the first wave on the predictor variable at the first wave in the OVERALL model statement to generate the main effects. Job satisfaction at the second wave was then regressed on job satisfaction at the first wave within the specific model statements for g – 1 of the predictor variable’s g levels to generate the interaction terms.
Results
Latent Transition Analysis: Job Importance
The three-wave, five-class LTA for job importance had high stability with 94 of the 100 random starts taken to completion returning the best solution (aBIC = 2,555,337.238) and an entropy of 0.758. The item-response probabilities and class prevalences, shown in table 2, are similar to those from Fritz (2022b: table 4) and resulted in the same five job importance classes: (1) Everything Is Very Important, (2) Challenge and Independence Are More Important than Salary and Benefits, (3) Benefits and Salary Are More Important than Responsibility, (4) Everything Is Somewhat Important, and (5) Advancement, Security, and Benefits Are Unimportant. Note that because the item-response probabilities were constrained to be equal across cycles, only one set of values is reported.
Item-response probabilities for the five-class job importance latent transition analysis solution with three response options: 2015, 2017, and 2019
Note(s):
Item-response probabilities were estimated simultaneously using the 2015, 2017, and 2019 Survey of Doctorate Recipients cycles but were constrained to be equal across cycles. Rows may not sum to 1.000 due to rounding. Response probabilities greater than or equal to 0.500 are considered salient and are represented in bold. Response probabilities more than twice as large as the next-largest probability in a row are highlighted in blue.
Source(s):
National Center for Science and Engineering Statistics, Survey of Doctorate Recipients.
The class transition probabilities were not constrained to be equal, so there are two sets of transition probabilities for job importance, as shown in table 3—one set for 2015 to 2017 and a second set for 2017 to 2019. Given that the largest difference between the 2015 to 2017 and 2017 to 2019 sets is 0.032, however, only the 2015 to 2017 transition probabilities are interpreted here. In interpreting the 2015 to 2017 transition probabilities, there are three issues to consider. First, the transition probabilities on the main diagonal, highlighted in bold, represent the probabilities of being in the same classes in 2017 as in 2015 (i.e., not transitioning to a new class), which is why they are often referred to as class stability parameters. Second, if there is absolutely no relationship between job importance class membership in both 2015 and 2017, then all of the transition probabilities should be equal to one out of five or 0.200. Third, Mplus provides statistical tests of whether the probability of transitioning to a specific class is different than the probability of staying in the same class (i.e., comparing the class stability parameter to each of the other transition probabilities in the same row). Mplus does not, however, provide statistical tests of whether any of the transition probabilities differ from 0.200 or from any of the other transition probabilities in the table, so any other differences of note will be discussed descriptively, not statistically.
Transition probabilities for the five-class job importance latent transition analysis: 2015, 2017, and 2019
SDR = Survey of Doctorate Recipients.
Note(s):
Transition probabilities in this table represent the probability of belonging to a specific job importance class at a given SDR cycle (column) conditional on belonging to a specific job importance class at the previous SDR cycle (row). Transition probabilities on the main diagonal represent the probability of being in the same class at both SDR cycles (i.e., class stability parameters) and are highlighted in bold. Rows may not sum to 1.000 due to rounding.
Source(s):
National Center for Science and Engineering Statistics, Survey of Doctorate Recipients.
All of the 2015 to 2017 transition probabilities differ statistically from the class stability parameter in the same row, meaning that individuals in each job importance class are most likely to remain in their 2015 class in 2017. Using Fritz 2022a: table 8, which provides differences in proportions for different effect sizes based on Cohen’s (1988) h, the class stability parameters exceed 0.200 by more than 0.378, meaning the probability of an individual being in the same class in 2017 as they were in 2015 is a large effect. That said, there is considerable movement between classes over time—between 26.3% and 39.9% of a class’s 2015 membership transitioned to a new class in 2017, which is a medium to large effect. These class stability values are similar to those found in other LTA studies such as Bray, Lanza, and Collins (2010), who found class stability parameters that ranged from 0.520 to 0.791 for alcohol use classes.
In investigating the probabilities of transitioning to new classes in 2017 (i.e., the off-diagonal transition probabilities), the smaller transition probabilities are in many ways more informative than the larger ones. For example, members of the Everything Is Very Important class in 2015 have only a 1.4% probability of transitioning to the Advancement, Security, and Benefits Are Unimportant class and only a 2.3% probability of transitioning to the Everything is Somewhat Important class in 2017, compared with 11.0% and 12.5% probabilities of transitioning to the Challenge and Independence Are More Important than Salary and Benefits class and the Benefits and Salary Are More Important than Responsibility class, respectively. Using Fritz 2022a: table 8, these are small effects. However, a member of the Everything Is Very Important class in 2015 who transitions to a new class in 2017 is approximately four and a half (Class 2 vs. Class 4) to nine times (Class 3 vs. Class 5) as likely to transition to a class that still considers at least some of the job factors as very important than a class that does not consider any of the factors to be very important.
Looking at the other rows in table 3, members of the Challenge and Independence Are More Important than Salary and Benefits class in 2015 who transition are more than twice as likely to move to the Everything Is Very Important class than any of the other classes. Members of the Benefits and Salary Are More Important than Responsibility class in 2015 who transition have a 15.7% probability of moving to the Everything Is Very Important class in 2017 but only a 1.9% chance of transitioning to the Advancement, Security, and Benefits Are Unimportant class. Members of the Everything Is Somewhat Important class in 2015 who transition are approximately two to three times more likely to move to one of the classes that rates some of the job factors as very important in 2017 (Classes 2 and 3), compared with the class that rates all of the job factors as very important or any of the job factors as unimportant (Classes 1 and 5). Finally, members of the Advancement, Security, and Benefits Are Unimportant class in 2015 who transition are most likely to move to the Challenge and Independence Are More Important than Salary and Benefits class and least likely to move to the Benefits and Salary Are More Important than Responsibility class, compared with moving to any of the other classes.
Latent Transition Analysis: Job Satisfaction
The three-wave, five-class LTA for job satisfaction had good stability with 71 of the 100 random starts returning the best solution (aBIC = 2,527,810.164) and an entropy of 0.726, although both were lower than for the job importance LTA. As with job importance, the job satisfaction item-response probabilities and class prevalences, shown in table 4, matched closely to those from the LCAs conducted by Fritz (2022b: table 6) and resulted in the same five job satisfaction classes: (1) Very Satisfied with Independence, Challenge, and Responsibility, (2) Very Satisfied with Everything, (3) Very Satisfied with Benefits, (4) Dissatisfied with Opportunities for Advancement, and (5) Somewhat Satisfied with Everything.
Item-response probabilities for the five-class job satisfaction latent transition analysis solution with three response options: 2015, 2017, and 2019
Note(s):
Item-response probabilities were estimated simultaneously using the 2015, 2017, and 2019 Survey of Doctorate Recipients cycles but were constrained to be equal across cycles. Rows may not sum to 1.000 due to rounding. Response probabilities greater than or equal to 0.500 are considered salient and are represented in bold. Response probabilities more than twice as large as the next-largest probability in a row are highlighted in blue.
Source(s):
National Center for Science and Engineering Statistics, Survey of Doctorate Recipients.
The class transition probabilities for job satisfaction are shown in table 5. As with job importance, the two sets of transition probabilities were quite similar (the largest difference was 0.033), so the interpretation will focus on the 2015 to 2017 transition probabilities. Also similar to the job importance transition probabilities, all of the 2015 to 2017 job satisfaction transition probabilities were statistically different than the class stability parameter in the same row. Again, these were all large effects and indicated that the most likely class membership for doctoral recipients in 2017 was the job satisfaction class to which they belonged in 2015. However, a slightly larger range of individuals transitioned to new classes, with between 24.1% and 40.3% of 2015 class members transitioning to a new class in 2017.
Transition probabilities for the five-class job satisfaction latent transition analysis: 2015, 2017, and 2019
SDR = Survey of Doctorate Recipients.
Note(s):
Transition probabilities in this table represent the probability of belonging to a specific job satisfaction class at a given SDR cycle (column) conditional on belonging to a specific job satisfaction class at the previous SDR cycle (row). Transition probabilities on the main diagonal represent the probability of being in the same class at both SDR cycles (i.e., class stability parameters) and are highlighted in bold. Rows may not sum to 1.000 due to rounding.
Source(s):
National Center for Science and Engineering Statistics, Survey of Doctorate Recipients.
Investigating the off-diagonal transition probabilities, it is interesting how members of the Dissatisfied with Opportunities for Advancement and the Somewhat Satisfied with Everything classes in 2015 transition compared with members of the other three classes. Individuals who transitioned into the Very Satisfied with Everything class in 2017 were approximately three to four times as likely to have been members of the Very Satisfied with Benefits class (0.166) in 2015, compared with the Somewhat Satisfied with Everything (0.052) or Dissatisfied with Opportunities for Advancement classes (0.042). The opposite effect was seen for individuals who transitioned to the Very Satisfied with Independence, Challenge, and Responsibility class who were more likely than the other two classes (Classes 2 and 3) to come from the Somewhat Satisfied with Everything (0.170) or Dissatisfied with Opportunities for Advancement classes (0.134).
Perhaps the most notable results are that individuals who transitioned into the Dissatisfied with Opportunities for Advancement class were more likely to belong to the Somewhat Satisfied with Everything class (0.113) in 2015 than to any of the other classes, whereas individuals who transitioned into the Somewhat Satisfied with Everything class were almost equally likely to have been members of the Very Satisfied with Independence, Challenge, and Responsibility class (0.109) or the Dissatisfied with Opportunities for Advancement class (0.126) in 2015. These results mean that individuals who transitioned into the dissatisfied class in 2017 were more likely to have transitioned from a class where they reported not being very satisfied with any of the nine job factors in their job in 2015 than a class where members were very satisfied with at least one job factors. It also means that the Somewhat Satisfied with Everything class is potentially an important intermediate step between belonging to one of the three classes whose members were very satisfied with at least some of the factors of their current job (Classes 1, 2, and 3) and the dissatisfied class (Class 5). That is, individuals who transitioned to the Somewhat Satisfied with Everything class in 2017 were more than twice as likely to then transition to the Dissatisfied with Opportunities for Advancement class in 2019 (0.118) than to transition to the Very Satisfied with Everything class (0.052).
Associative Latent Transition Analysis Model
The 2015 to 2017 ALTA model for job satisfaction with job importance class at 2015 predicting the transitions (image 2, part C) produced five separate sets of 25 job satisfaction class transition probabilities as shown in table 6. As with the previous LTA results, the main diagonal of the transition probability matrix for each 2015 job importance class is the class stability parameters (in bold), and the off-diagonals are the probabilities of transitioning to specific job satisfaction classes in 2017 (columns) given membership in both a specific job satisfaction classes in 2015 (rows) and a specific 2015 job importance class. The chi-squared difference test between the model with the interaction terms constrained to be zero (L0 = -1451652.566, s0 = 0.9981, p0 = 60) and the model with the interaction terms freely estimated (L1 = -1451580.136, s1 = 1.0000, p1 = 124) was statistically significant at the 0.001 level (χ2ΔSB(64) = 144.602, p < 0.001). This information means that one or more transition probabilities differ between job importance classes. Although the layout of table 6 makes sense conceptually, the purpose of estimating this model is to examine differences in transition probabilities for job satisfaction across the job importance classes. This scenario is more easily accomplished by reordering the values in table 6 so that the corresponding rows of transition probabilities for each job importance class are grouped together as in table 7. Note that the 2017 to 2019 ALTA model was also run, but the results were similar to the results of the 2015 to 2017 model with an average difference in transition probabilities of only 0.021, although one difference did exceed 0.10. Given the small average difference between the 2015 to 2017 and 2017 to 2019 ALTA models, and the fact that the models were run separately so that the 2017 to 2019 model had more missing data due to attrition, only the 2015 to 2017 model results are reported and interpreted.
Transition probabilities for the 2015 to 2017 job satisfaction and job importance ALTA model by job importance class
ALTA = associative latent transition analysis; SDR = Survey of Doctorate Recipients.
Note(s):
Transition probabilities in this table represent the probability of belonging to a specific job satisfaction class at the 2017 SDR cycle (column) conditional on belonging to a specific job satisfaction class at the 2015 SDR cycle (row), grouped by job importance class at the 2015 SDR cycle. Class stability parameters are in bold. Rows may not sum to 1.000 due to rounding.
Source(s):
National Center for Science and Engineering Statistics, Survey of Doctorate Recipients.
Examining table 7, the primary pattern that emerges is a difference in the job satisfaction class stability parameters between the Everything Is Somewhat Important class and the Advancement, Security, and Benefits Are Unimportant class. For example, members of the Very Satisfied with Independence, Challenge, and Responsibility class in 2015 who were also members of the Advancement, Security, and Benefits Are Unimportant class in 2015 were least likely to transition to a new job satisfaction class in 2017 (0.763) compared to members of the Very Satisfied with Independence, Challenge, and Responsibility class in 2015 who were also members of the Everything Is Somewhat Important class in 2015 were most likely to transition to a different job satisfaction class in 2017 (0.619), a difference of 0.144 (highlighted in blue). Because every person who transitions must move into a new class, the decreased stability for people who were very satisfied with their independence and viewed all job factors as somewhat important means the probability of transitioning into one or more of the other job satisfaction classes must increase. The results show exactly this case, with the probability of members of the Very Satisfied with Independence, Challenge, and Responsibility class in 2015 who were also members of the Everything Is Somewhat Important class in 2015 having a probability of transitioning to the Somewhat Satisfied with Everything class in 2017 of 0.257. This scenario is over four times greater than for members of the Advancement, Security, and Benefits Are Unimportant class (0.061) and over twice as high as for the entire sample (0.109). This effect is especially important given the LTA results in table 5, which shows members of the Somewhat Satisfied with Everything class who transitioned were most likely to transition to the Very Satisfied with Independence, Challenge, and Responsibility and Dissatisfied with Opportunities for Advancement classes.
Similar but opposite effects are seen for two of the other 2015 job satisfaction classes. Members of the Very Satisfied with Benefits and the Somewhat Satisfied with Everything classes in 2015 who were also members of the Advancement, Security, and Benefits Are Unimportant class in 2015 were more likely to transition (stability estimates were 0.577 and 0.459, respectively) than were members of either of those two 2015 job satisfaction classes who were also members of the Everything Is Somewhat Important class in 2015 (stability estimates were 0.755 and 0.636, respectively). Members of the Very Satisfied with Benefits class in 2015 who were also members of the Advancement, Security, and Benefits Are Unimportant class in 2015 were approximately four to six times more likely to transition to the Very Satisfied with Independence, Challenge, and Responsibility class in 2017 (0.124) than to any other job satisfaction class. Members of the Somewhat Satisfied with Everything class in 2015 who were also members of the Advancement, Security, and Benefits Are Unimportant class in 2015 had an approximately one in three chance (0.297) of transitioning to the Very Satisfied with Independence, Challenge, and Responsibility class in 2017, higher than transitioning to any other job satisfaction class. What is even more remarkable, however, is that the class stability parameter for the somewhat satisfied individuals who thought advancement was unimportant is the only one less than 0.500 (0.459) in table 7, meaning these were the only doctorate recipients who were more likely to transition to a new job satisfaction class in 2017 than remain in their existing 2015 job satisfaction class.
Transition probabilities for the 2015 to 2017 job satisfaction and job importance ALTA model by 2015 job satisfaction class
ALTA = associative latent transition analysis; SDR = Survey of Doctorate Recipients.
Note(s):
Transition probabilities in this table represent the probability of belonging to a specific job satisfaction class at the 2017 SDR cycle (column) conditional on belonging to a specific job importance class at the 2015 SDR cycle (row), grouped by job satisfaction class at the 2015 SDR cycle. The 2015 to 2017 transition probabilities for the entire sample from the job satisfaction latent transition analysis in table 5 are provided for reference and highlighted in gray. Class stability parameters are in bold. Largest difference in class stability parameters for each 2015 satisfaction class, except Class 2, and the corresponding opposite effect are highlighted in blue. Rows may not sum to 1.000 due to rounding.
Source(s):
National Center for Science and Engineering Statistics, Survey of Doctorate Recipients.
The largest difference in stability parameters for members of the Dissatisfied with Opportunities for Advancement class in 2015 is between the Challenge and Independence Are More Important than Salary and Benefits (0.561) and the Everything Is Somewhat Important (0.660) classes, a difference of 0.099, which is just above the threshold for a small effect. The only 2015 satisfaction class for which job importance class membership seems to have no effect is the Very Satisfied with Everything class, whose largest stability parameter difference is only 0.043 and therefore too small to be considered a meaningful effect. However, this statistic could also be an artifact of this class having a higher percentage of rapid responders (see Fritz 2022a for more information).
Latent Transition Analysis Models with Covariates
Given the similarities between the 2015 to 2017 and 2017 to 2019 ALTA model results for job importance and the issues with attrition for the 2017 to 2019 model, only the impact of the covariates on the 2015 to 2017 job satisfaction class transitions was investigated. The Satorra-Bentler chi-square difference tests were nonsignificant at the 0.001 level for degree area (χ2ΔSB (32) = 53.002, p = 0.011), whether the doctoral recipient was living in the United States during the week of 1 February 2015 (χ2ΔSB (16) = 15.685, p = 0.475), and whether recipients identified as having a physical disability (χ2ΔSB (16) = 30.950, p = 0.014). This scenario means that the probability of transitioning to a new job satisfaction class or remaining in the same job satisfaction class between the 2015 and 2017 SDR cycles did not depend on these three variables, so these results are not presented or discussed further. The Satorra-Bentler test for self-reported gender was statistically significant (χ2ΔSB(16) = 45.797, p < 0.001), but none of the interaction terms from the loglinear model reached significance. In addition, an examination of the transition probabilities (not shown here) showed an average difference of only 0.017 between males and females, with the largest being only 0.043, which fails to reach the level of a small effect. Therefore, despite statistical significance, the interaction effect of gender is considered negligible and will not be discussed further.
Employment Sector
The Satorra-Bentler test for employment sector was statistically significant (χ2ΔSB (32) = 153.661, p < 0.001). The significance of this test means that one or more job satisfaction class transition probabilities, shown in table 8, are different depending on whether the doctoral recipient’s job in 2015 was in education, government, or business and industry. Note that only doctoral recipients who were employed in 2015 were included in this model because Mplus will not allow missing values on a covariate entered using the KNOWNCLASS statement, and these individuals do not provide any information about change in job satisfaction class between 2015 and 2017 because they were not asked to complete the job satisfaction questions for the 2015 SDR cycle. Although there are many differences in table 8, only three reach the level of a small effect and, unlike job importance, none of the effects are related to the class stability parameters. Instead, members of the Dissatisfied with Opportunities for Advancement or Somewhat Satisfied with Everything classes in 2015 who were also working in the education sector in 2015 were less likely to transition to the Very Satisfied with Benefits class in 2017 (0.045 and 0.041, respectively), compared with members of those classes who worked in government (0.148 and 0.096, respectively) or business and industry (0.109 and 0.104, respectively). In addition, members of the Very Satisfied with Everything class in 2015 were less likely to transition to the Very Satisfied with Independence, Challenge, and Responsibility class in 2017 if they worked in government in 2015 (0.034), compared with those who worked in business and industry (0.087).
Transition probabilities for the 2015 to 2017 job satisfaction LTA model with covariates for employment sector by 2015 job satisfaction class
LTA = latent transition analysis; SDR = Survey of Doctorate Recipients.
Note(s):
Transition probabilities in this table represent the probability of belonging to a specific job satisfaction class at the 2017 SDR cycle (column) conditional on belonging to a specific employment sector at the 2015 SDR cycle (row), grouped by 2015 job satisfaction class. The 2015 to 2017 transition probabilities for the entire sample from the job satisfaction LTA in table 5 are provided for reference and highlighted in gray. Class stability parameters are in bold. Differences reaching the level of a small effect or larger are highlighted in blue. Rows may not sum to 1.000 due to rounding.
Source(s):
National Center for Science and Engineering Statistics, Survey of Doctorate Recipients.
Race and Ethnicity
The Satorra-Bentler test for self-reported race and ethnicity (χ2ΔSB (64) = 195.290, p < 0.001) was also statistically significant. Examining the transition probabilities in table 9, numerous differences reach the level of a small effect. One trend indicates that the same difference between participants who identified as Asian and those who identified as White is seen for the members of the three job satisfaction classes who reported being very satisfied with at least one factor of their current job. That is, members of the Very Satisfied with Independence, Challenge, and Responsibility class, the Very Satisfied with Everything class, and the Very Satisfied with Benefits class in 2015 who identified as White were more likely to remain in the same class in 2017 (0.731, 0.795, and 0.721, respectively) than members of those classes who identified as Asian (0.641, 0.659, and 0.606, respectively). In turn, members of these three classes in 2015 who transitioned to new classes in 2017 and identified as Asian were two to four times more likely to transition to the Somewhat Satisfied with Everything class (0.198, 0.094, and 0.115, respectively), compared with members of those classes who identified as White (0.094, 0.023, and 0.041, respectively). Doctorate recipients who identified as Asian and were members of the Dissatisfied with Opportunities for Advancement class in 2015 who transitioned to a new job satisfaction class in 2017 also had the highest probability of transitioning to the Somewhat Satisfied with Everything class (0.211) and the highest probability of remaining in the Somewhat Satisfied with Everything class (0.646), compared with the other four race and ethnicity categories. Although these are small effects, Fritz (2022a) also found that doctorate recipients who identified as Asian made up a larger percentage of membership of the 2017 Somewhat Satisfied with Everything class (and Whites made up a smaller percentage), compared with the other job satisfaction classes.
A second trend shown is the difference between doctorate recipients who identify as Asian, Black, Hispanic, and White, compared with members of the “other” group. The largest difference in table 9, which reaches the level of a medium effect, is that in the stability parameters for members of the Somewhat Satisfied with Everything class in 2015 who identified as other (0.378) compared to those who identified as Asian (0.646), with the differences dropping to small effects when comparing the other race and ethnicity category with the other three non-Asian race and ethnicity categories. These statistics mean that persons who reported other race and ethnicity and were in the Somewhat Satisfied with Everything class in 2015 had a 62.2% probability (i.e., 1 - 0.378) of transitioning to a new job satisfaction class in 2017, with the highest probability of transitioning being to the Dissatisfied with Opportunities for Advancement class (0.210) and a relatively small probability of transitioning to the Very Satisfied with Everything class (0.037). In addition, members of the other race and ethnicity category who were members of the Very Satisfied with Benefits class in 2015 were most likely to transition to a new class in 2017 (i.e., 1 - 0.589 = 0.411) and members of the other race and ethnicity category who were also members of the Very Satisfied with Everything class in 2015 were least likely to transition to the Very Satisfied with Independence, Challenge, and Responsibility class in 2017 (0.023) than were members of the other four race and ethnicity categories.
Transition probabilities for the 2015 to 2017 job satisfaction LTA model with covariates for race and ethnicity by 2015 job satisfaction class
LTA = latent transition analysis; SDR = Survey of Doctorate Recipients.
Note(s):
Transition probabilities in this table represent the probability of belonging to a specific job satisfaction class at the 2017 SDR cycle (column) conditional on self-reported race and ethnicity at the 2015 SDR cycle (row), grouped by job satisfaction class at the 2015 SDR cycle. The 2015 to 2017 transition probabilities for the entire sample from the job satisfaction LTA in table 5 are provided for reference and highlighted in gray. Class stability parameters are in bold. Select cells discussed in the text are highlighted in blue. Rows may not sum to 1.000 due to rounding.
Source(s):
National Center for Science and Engineering Statistics, Survey of Doctorate Recipients.
Discussion
Conclusions and Future Directions
As discussed in the introduction, LTA and its variations considered in this working paper expanded on the LCA results from Fritz (2022a, 2022b) by testing longitudinal hypotheses regarding how individuals transition between job satisfaction and job importance classes. For example, the LTAs revealed that on average 32.1% of the members of a job satisfaction class at one SDR cycle transitioned to a different job satisfaction class at the next SDR cycle. Although this result may seem relatively stable given that over two-thirds of doctoral degree holders remain in the same job satisfaction class at consecutive SDR cycles, fewer than half (46.1%; 0.679 × 0.679) were estimated to have been in the same job satisfaction class across all three SDR cycles, although some of these individuals transitioned back to their original 2015 class in 2019. What is important, however, is less whether an individual transitions to a different class and more being able to predict the class to which they transition. Specifically, the most notable finding is that members of the Dissatisfied with Opportunities for Advancement class were more likely to have come from the Somewhat Satisfied with Everything class than any of the other classes. When paired with the finding that individuals who transitioned into the Somewhat Satisfied with Everything class were almost equally likely to have come from the Dissatisfied with Opportunities for Advancement class as the Very Satisfied with Independence, Challenge, and Responsibility class, this scenario presents three important conclusions.
First, these results reveal a pathway by which doctoral degree holders may enter the Dissatisfied with Opportunities for Advancement class. That is, members of the Very Satisfied with Independence, Challenge, and Responsibility class in 2015 who transitioned were most likely to become members of the Somewhat Satisfied with Everything class in 2017. In turn, members of the Somewhat Satisfied with Everything class in 2017 who transitioned had only a slightly lower chance of moving to the Dissatisfied with Opportunities for Advancement class in 2019 as transitioning back to the Very Satisfied with Independence, Challenge, and Responsibility class. Given that Fritz (2022a) found the Very Satisfied with Independence, Challenge, and Responsibility class had a significantly higher percentage of members with jobs in the education sector, compared with the other four job satisfaction classes, this pathway may be especially relevant for academic researchers. That is, academic researchers who transition to the Somewhat Satisfied with Everything class and later transition to the Dissatisfied with Opportunities for Advancement class may find their next transition is to a nonacademic career. Therefore, future research could focus on developing a better understanding of what causes academic members of the Very Satisfied with Independence, Challenge, and Responsibility class to transition to the Somewhat Satisfied with Everything class, and it could also focus on what causes members of the Somewhat Satisfied with Everything class to transition to the Dissatisfied with Opportunities for Advancement class versus back to the Very Satisfied with Independence, Challenge, and Responsibility class. The responses to these queries would provide insight into what steps could be taken to prevent these individuals from becoming dissatisfied.
The second conclusion from the results is that individuals in the Dissatisfied with Opportunities for Advancement class were more likely to transition than were members of the three classes who were very satisfied with at least one job factor. At first glance, this notion seems like a positive: if these dissatisfied individuals simply transition to the Somewhat Satisfied with Everything class and back to the Dissatisfied with Opportunities for Advancement class, however, the dissatisfied individuals are becoming less dissatisfied (at least for a time) but are not actually reaching the level of being highly satisfied. Instead, the divide seems to be one of individuals whose pattern of responses includes being highly satisfied with one or more of the factors of their current job and individuals whose pattern of responses does not include being highly satisfied with any of the factors of their current job. This idea is illustrated by the results in table 5, which show that individuals who transitioned into the Dissatisfied with Opportunities for Advancement class in 2017 were most likely to have been members of the Somewhat Satisfied with Everything class in 2015 (0.113) and least likely to have been members of the Very Satisfied with Everything class in 2015 (0.014). Of course, it is also possible that these individuals’ transition from the Dissatisfied with Opportunities for Advancement class is caused by them starting a new job between the 2015 and 2017 SDR cycles. Future research could focus on whether becoming highly satisfied with any single job factor is enough to prevent an individual from becoming dissatisfied or whether the high level of satisfaction needs to be with a specific job factor or set of job factors (e.g., salary vs. salary and benefits) to prevent transitioning into the dissatisfied class.
The final take-away is that the Somewhat Satisfied with Everything class really seems to be very important and not just a residual class that improves both model fit and the separation of the other classes as originally hypothesized by Fritz (2022a, 2022b). In addition to its potential intermediary role in the pathway from the Very Satisfied with Independence, Challenge, and Responsibility class to the Dissatisfied with Opportunities for Advancement class, individuals who identified as Asian had the highest probability of transitioning into the Somewhat Satisfied with Everything class. Although Asian members of the Somewhat Satisfied with Everything class do not have a higher probability of then transitioning to the Dissatisfied with Opportunities for Advancement class than the other racial and ethnic groups, they did have the highest probability of remaining in the Somewhat Satisfied with Everything class. These differences are only small effects, but they still seem quite important. Future research could focus on better understanding whether (and if so, which) cultural and contextual factors explain membership in the Somewhat Satisfied with Everything class, not just for individuals who identify as Asian, but for all doctoral degree holders.
Limitations
Despite the large sample and longitudinal person-centered methods, this working paper has several limitations. First, although LTA and ALTA are very powerful and flexible models, they do present challenges. The software to estimate these models has advanced tremendously over the past decade, but figuring out how to specify these models remains nontrivial, as is access to adequate computational resources to estimate the model. As illustrated in these results, many tough decisions regarding the data and the model specification had to be made in order to get the models to run within a reasonable amount of time (i.e., days instead of weeks). Even if they could be run successfully, loglinear models present challenges for parameter estimate interpretation and specific effects of interest testing because these are output in the logit metric, but it is generally more desirable to discuss the results in terms of probabilities.
The second limitation is ALTA models that included all three SDR cycles and additional variables were not considered here. For example, Fritz (2022a) showed that the Advancement, Security, and Benefits are Unimportant class had a higher percentage of older individuals than the other four importance classes for the 2017 cycle, so including a three-way interaction between job satisfaction class, job importance class, and age could have produced interesting results. Similarly, the ALTA models fit here did not consider whether or when individuals changed jobs, which is almost certainly related to job satisfaction class but is not identical. Plenty of people who hate their jobs stay in them, and many people who love their current jobs take new jobs. Although these factors were partially due to computational limitations, it is also caused by the structure of the SDR itself. Despite repeatedly gathering information on the same individuals, the SDR only provides biannual cross-sectional snapshots of doctoral recipients’ employment and job satisfaction. A more dynamic temporal design would be necessary to understand how job satisfaction class relates to the who, why, and when of movement between jobs, employers, and employment sectors.
The final limitation is that while the LTA and ALTA models can be used to test longitudinal hypotheses of interest, the estimated effects are not necessarily causal. That is, the use of longitudinal data meets the requirement of temporal precedence, but the data are strictly observational and therefore are likely to violate many of the other assumptions necessary to make claims of causality (Murnane and Willett 2011). Although the use of random assignment seems untenable in the SDR, the use of quasi-experimental designs such as nonequivalent control groups or regression discontinuity designs to study causal effects relating to movement among job satisfaction classes may be considered to positive effect (Shadish, Cook, and Campbell 2002). For example, one option would be collecting more data on members of the Dissatisfied with Opportunities for Advancement class and comparing those who did change jobs between cycles to those who did not (i.e., a nonequivalent control group).
References
Agresti A. 2013. Categorical Data Analysis. 3rd ed. Hoboken, NJ: Wiley.
Bray BC, Lanza ST, Collins LM. 2010. Modeling Relations Among Discrete Developmental Processes: A General Approach to Associative Latent Transition Analysis. Structural Equation Modeling: A Multidisciplinary Journal 17:541–69. Available at https://www.tandfonline.com/doi/abs/10.1080/10705511.2010.510043.
Cohen J. 1988. Statistical Power Analysis for the Behavioral Sciences. 2nd ed. Mahwah, NJ: Lawrence Erlbaum Associates. Available at https://www.utstat.toronto.edu/~brunner/oldclass/378f16/readings/CohenPower.pdf.
Collins LM, Lanza ST. 2010. Latent Class and Latent Transition Analysis: With Applications in the Social, Behavioral, and Health Sciences. Hoboken, NJ: Wiley.
Flaherty BP. 2008. Examining Contingent Discrete Change over Time with Associative Latent Transition Analysis. In Hancock GR, Samuelsen, KM, editors, Advances in Latent Variable Mixture Models, pp. 77–103. Charlotte, NC: Information Age Publishing, Inc.
Fritz MS; National Center for Science and Engineering Statistics (NCSES). 2022a. Job Importance versus Job Satisfaction: A Latent Class Analysis of the 2017 Survey of Doctorate Recipients. NCSES 22-207. Alexandria, VA: National Science Foundation. Available at https://ncses.nsf.gov/pubs/ncses22207/.
Fritz MS; National Center for Science and Engineering Statistics (NCSES). 2022b. Replicating the Job Importance and Job Satisfaction Latent Class Analysis from the 2017 Survey of Doctorate Recipients with the 2015 and 2019 Cycles. NCSES 22-208. Alexandria, VA: National Science Foundation. Available at https://ncses.nsf.gov/pubs/ncses22208/.
Hagenaars JA. 1993. Loglinear Models with Latent Variables. Newbury Park, CA: Sage.
Kaloudis A, Aspelund A, Koch PM, Lauvås TA, Tuft Mathisen M, Strand Ø, Sørheim R, Aadland T. 2019. How Universities Contribute to Innovation: A Literature Review-Based Analysis. Trondheim, Norway: Norwegian University of Science and Technology. Available at https://www.ntnu.no/documents/1272503658/1276139892/Rapport_How+universities+contribute+to+innovation_web.pdf/2233414b-d9cd-85ad-6bc8-58b9bc0c9d78?t=1574847934147.
Murnane RJ, Willett JB. 2011. Methods Matter: Improving Causal Inference in Educational and Social Science Research. New York, NY: Oxford University Press.
National Center for Science and Engineering Statistics (NCSES). 2018. Survey of Doctorate Recipients, Survey Year 2015. Alexandria, VA: National Science Foundation. Available at https://ncsesdata.nsf.gov/doctoratework/2015/.
National Center for Science and Engineering Statistics (NCSES). 2019. Survey of Doctorate Recipients, Survey Year 2017. Alexandria, VA: National Science Foundation. Available at https://ncsesdata.nsf.gov/doctoratework/2015/.
National Center for Science and Engineering Statistics (NCSES). 2021. Survey of Doctorate Recipients, 2019. NSF 21-320. Alexandria, VA: National Science Foundation. Available at https://ncses.nsf.gov/pubs/nsf21320/.
NORC at the University of Chicago. 2016. NORC Data Enclave. Available at: https://www.norc.org/PDFs/BD-Brochures/2016/Data Enclave One Sheet.pdf.
Odhiambo GO. 2013. Academic Brain Drain: Impact and Implications for Public Higher Education Quality in Kenya. Research in Comparative and International Education 8:510–23. Available at https://doi.org/10.2304/rcie.2013.8.4.510.
Ruiz Castro M, Van der Heijden B, Henderson EL. 2020. Catalysts in Career Transitions: Academic Researchers Transitioning into Sustainable Careers in Data Science. Journal of Vocational Behavior 122:Article 103479. Available at https://doi.org/10.1016/j.jvb.2020.103479.
Satorra A, Bentler PM. 2010. Ensuring Positiveness of the Scaled Difference Chi-square Test Statistic. Psychometrika 75:243–48. Available at https://doi.org/10.1007/s11336-009-9135-y.
Shadish WR, Cook TD, Campbell DT. 2002. Experimental and Quasi-Experimental Designs for Generalized Causal Inference. 2nd ed. Boston, MA: Houghton Mifflin Company.
Suggested Citation
Fritz MS, Bray BC; National Center for Science and Engineering Statistics (NCSES). 2023. Investigating the Stability of Job Importance and Job Satisfaction over Time: A Latent Transition Analysis of the Survey of Doctorate Recipients. NCSES 23-213. Alexandria, VA: National Science Foundation. Available at https://ncses.nsf.gov/pubs/ncses23213.
Contact Us
NCSES
National Center for Science and Engineering Statistics
Directorate for Social, Behavioral and Economic Sciences
National Science Foundation
2415 Eisenhower Avenue, Suite W14200
Alexandria, VA 22314
Tel: (703) 292-8780
FIRS: (800) 877-8339
TDD: (800) 281-8749
E-mail: ncsesweb@nsf.gov
Read more about the source:
Survey of Doctorate Recipients (SDR).